P values
- @Medicine.Daily
- Apr 12, 2020
- 2 min read
Updated: Jun 2, 2020
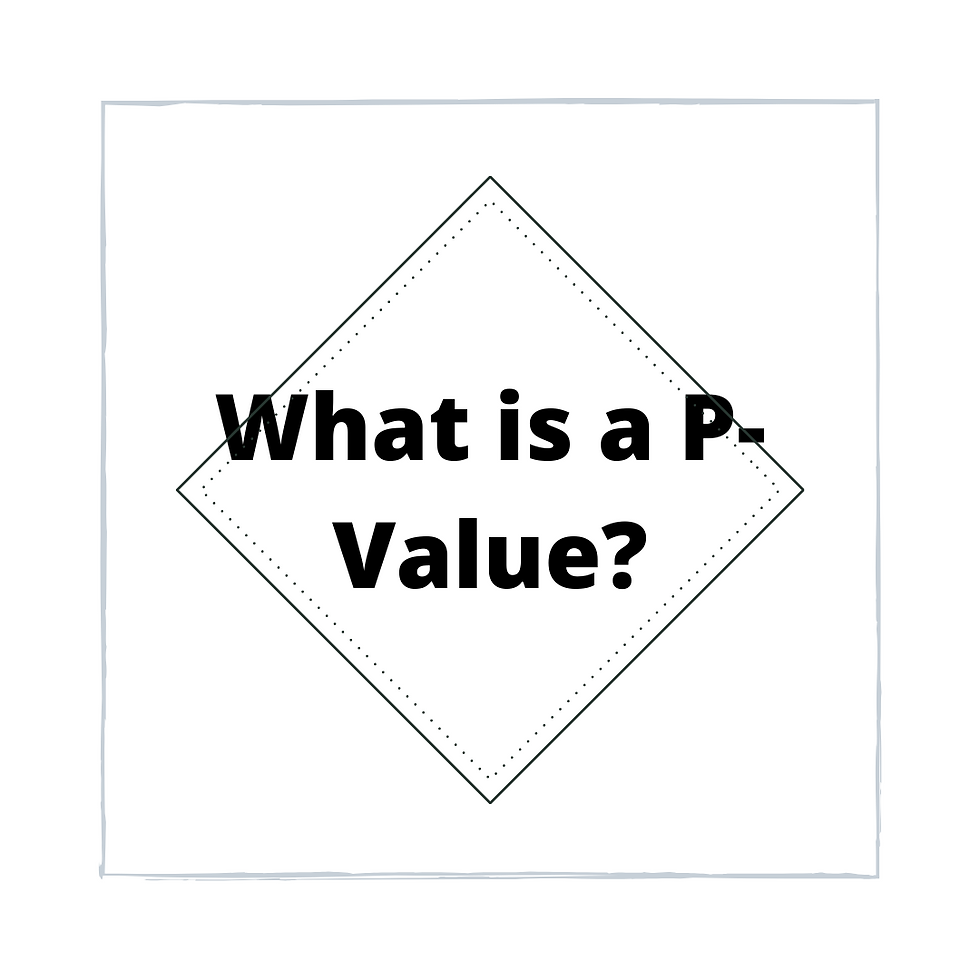
What is a P value? If you are starting out reading scientific journals or getting interested in science at school you’ve probably heard about this concept a bit already, but what does it mean?
When you want to do an experiment you want to test your idea, otherwise known as a hypothesis. Confusingly, we measure something called the “null hypothesis”. This is idea that in our experiment there will be no significant difference between the two things we are testing.
If we can use statistics to say that there IS a significant difference then we can REJECT the null hypothesis.
How do we do this?
P value! The probability value (p) is a number between 0 and 1 and we can set a value that tell us whether our date is significant or insignificant. The common cut off is:
P value <0.05 is a significant result. The smaller the P value the better.
P value >0.05 is an insignificant result.
Don’t forget – you can’t just take a P-value in isolation, it should be looked at in addition to other factors in particular confidence intervals
Why is the P-value flawed?
Firstly, remember we have the null hypothesis, the next part is to show that the P value is the probability of the same result if anyone did the experiment assuming the NULL hypothesis is TRUE (i.e no significance).
If the P is small, the outcome shows significance, right?
Not necessarily. As previously mentioned we all need to be robust when looking at research papers as sometimes people want to try and make data look more convincing. At the moment there is only really an incentive to publish positive findings.
Don’t forget, negative findings are really important too as they stop us repeating ourselves and can sometimes shed light on new things in the future!
Changing the p-value is sometimes called p-hacking which sounds a lot cooler than it actually is.
As opposed to P values being binary or black and white, significant or insignificant, we are supposed to look at them as areas of grey with graded significance.
It has previously been noted that the odds of rejecting the null hypothesis with a p value of 0.05 are only about 4 to 1 and that to truly get a rejected null we should really be looking at 0.005 or 0.001! A pretty massive distance.
The real take home from this is don’t just see “oh the p value is significant my job is done” but look at all the factors influencing it. In some circumstances even think a large p-value may have some significance in the rest of the data!
Comments